The Promise and Perils of Synthetic Data in AI Development
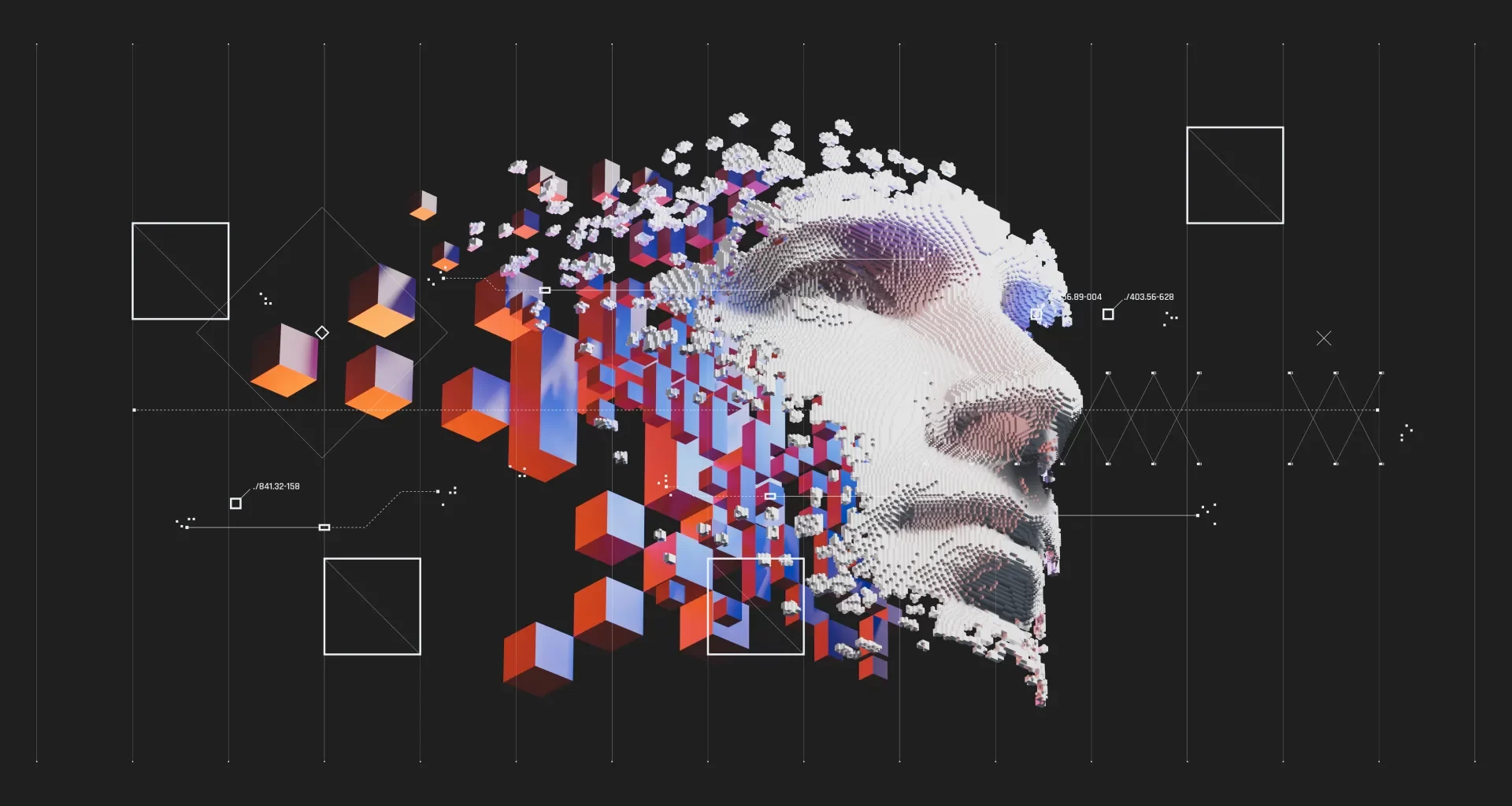
The Promise and Pitfalls of Synthetic Data in AI Development
AI depends on vast amounts of data, but sourcing high-quality, annotated datasets is becoming increasingly challenging. Synthetic data — generated by AI to simulate real-world data — is emerging as a potential solution, offering scalability and cost savings. While companies like Anthropic, Meta, and OpenAI are incorporating synthetic data into model training, this approach comes with risks and limitations.
Why AI Needs Data
AI systems are statistical machines trained to detect patterns from annotated data. High-quality annotations, such as labels in image datasets, are crucial for accurate predictions. However, creating such datasets is expensive, labor-intensive, and often biased due to human errors or limited representation.
The demand for labeled data has created a billion-dollar annotation industry, but even this has its limits. Data acquisition is becoming harder as copyright concerns and content restrictions grow. For example, over 35% of the world’s top websites now block OpenAI’s web scraper. Researchers predict that by 2026–2032, developers may face significant data shortages for training generative AI models.
The Rise of Synthetic Data
Synthetic data offers a scalable alternative, creating annotations and datasets without the constraints of real-world data collection. Companies like Writer, Microsoft, and Nvidia are using synthetic data to train models at a fraction of the cost. Writer’s Palmyra X 004 model, trained mostly on synthetic data, cost just $700,000 to develop compared to $4.6 million for similar models.
Synthetic data generation is becoming a lucrative industry, projected to be worth $2.34 billion by 2030. Analysts expect 60% of data used in AI and analytics projects this year to be synthetic. It also enables innovative use cases, such as Meta’s video captioning for training Movie Gen and OpenAI’s enhancements for ChatGPT’s Canvas feature.
The Challenges of Synthetic Data
Despite its promise, synthetic data is no silver bullet. It inherits biases from the models that generate it. For instance, poorly represented groups in the training data will remain underrepresented in the synthetic outputs.
Researchers from Rice University and Stanford found that over-reliance on synthetic data could degrade model quality over generations, leading to less diverse and less accurate outputs. Mixing real-world data into synthetic datasets can help mitigate this, but the risk of compounding errors remains.
Hallucinations — fabricated or nonsensical outputs from AI models — further complicate synthetic data’s reliability. Complex models like OpenAI’s o1 can generate synthetic data with subtle inaccuracies that are difficult to identify, potentially degrading the performance of models trained on them.
The Future of Synthetic Data
Synthetic data holds immense potential to address data shortages and reduce annotation costs, but it must be approached with caution. Blending real-world data, improving model transparency, and refining generation techniques are critical to maximizing its benefits while minimizing risks.
As AI continues to evolve, synthetic data will play a key role in shaping its trajectory, but careful oversight and innovation will be essential to unlocking its full potential.
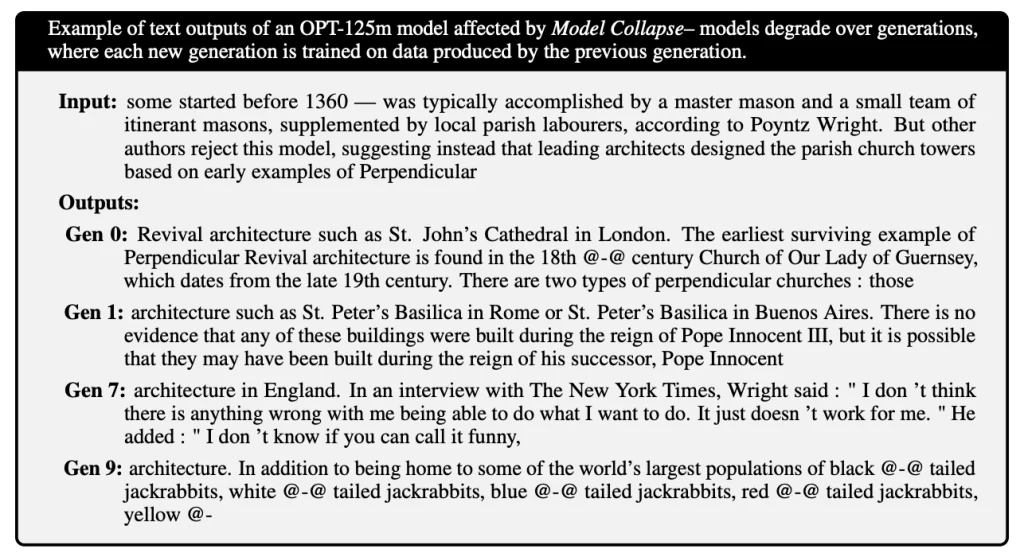
Image Credits:Ilia Shumailov et al.
A follow-up study shows that other types of models, like image generators, aren’t immune to this sort of collapse:
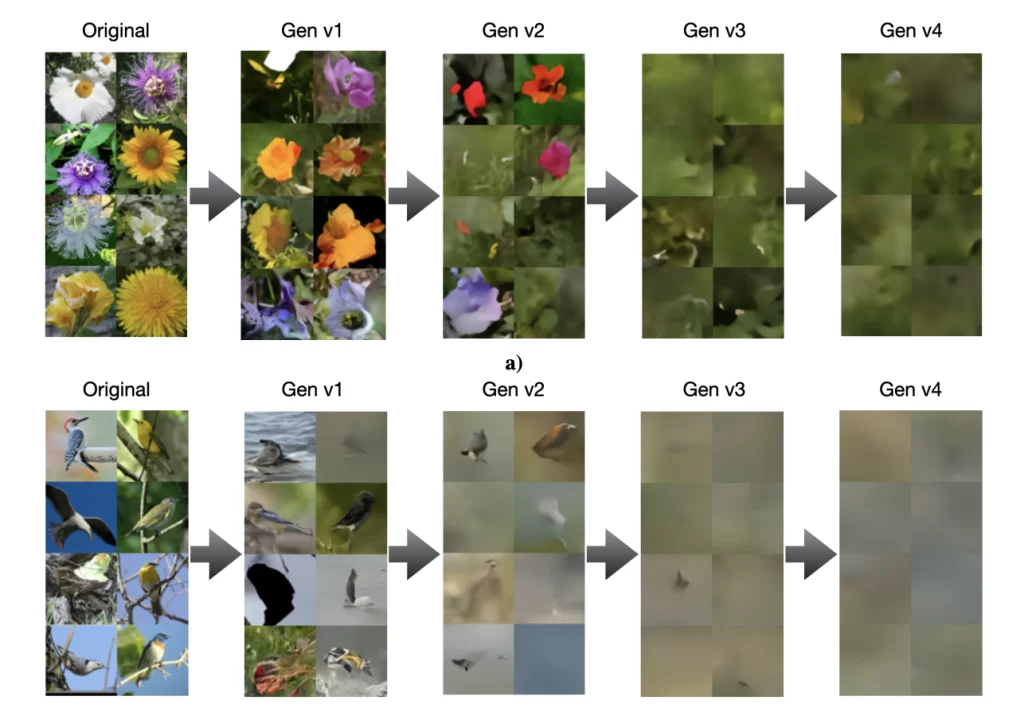
Image Credits:Ilia Shumailov et al.
Synthetic Data Needs Human Oversight to Avoid AI Model Collapse
While synthetic data offers promise as a scalable solution for training AI, experts like Luca Soldaini caution against relying on it unchecked. Without proper curation and supplementation with fresh, real-world data, synthetic data can lead to model collapse, where AI systems become less creative and more biased, compromising their functionality.
“Raw synthetic data isn’t to be trusted,” says Soldaini. “It must be thoroughly reviewed, curated, and filtered.” He emphasizes that researchers need to inspect generated data, refine the generation process, and implement safeguards to eliminate low-quality outputs. Synthetic data pipelines, he warns, are not inherently self-improving and require vigilant human oversight.
OpenAI CEO Sam Altman has speculated that AI might eventually produce synthetic data capable of training itself effectively. However, no major AI lab has yet achieved this, and no model trained entirely on synthetic data has been released.
For now, human involvement remains crucial to ensure AI models maintain accuracy and avoid degrading over time. Synthetic data, while valuable, is not a substitute for careful dataset management and human expertise.