Nvidia CEO Claims AI Chip Advancements Are Outpacing Moore’s Law
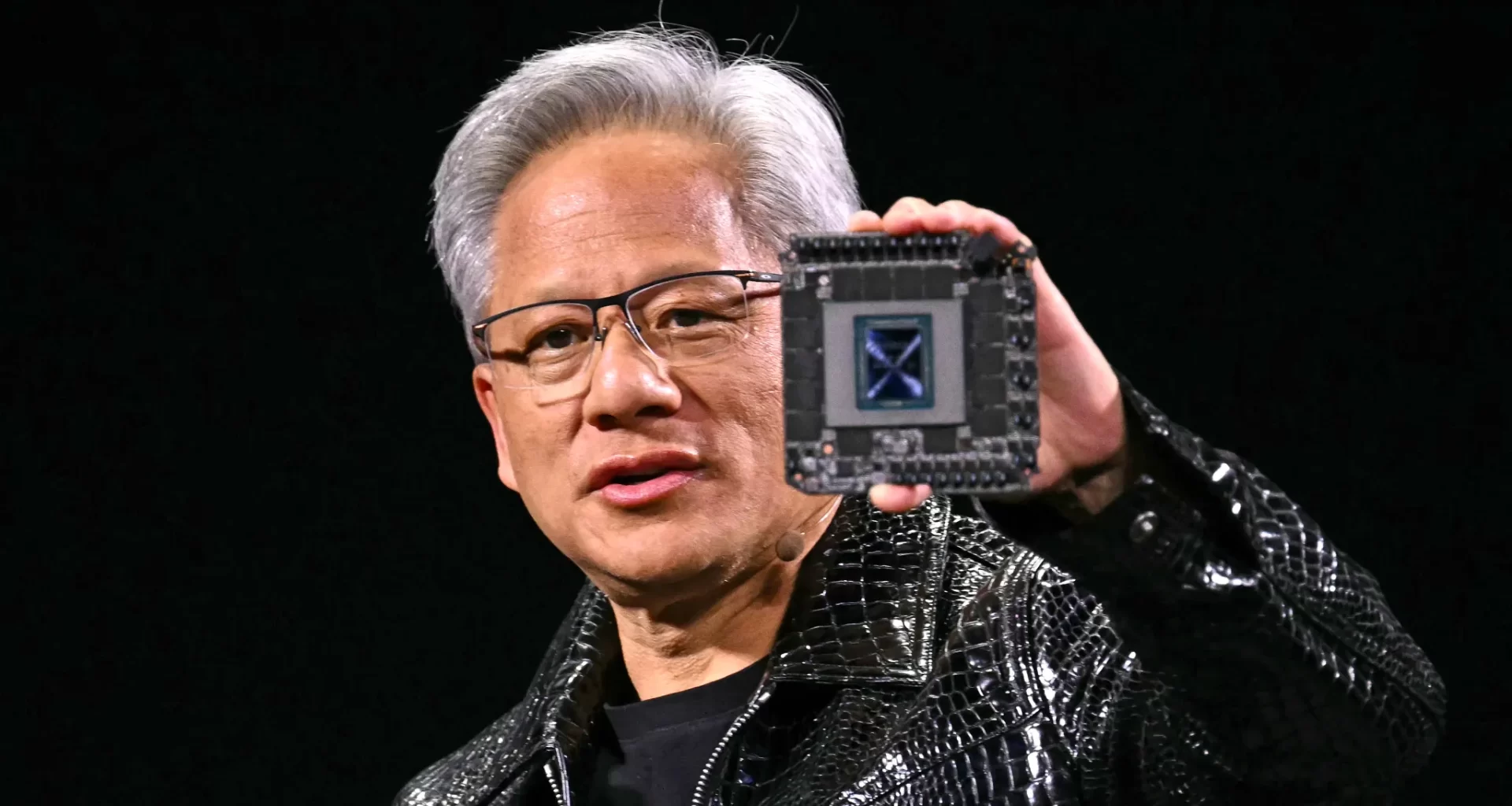
Nvidia CEO Claims AI Chip Progress Is Surpassing Moore’s Law
Nvidia CEO Jensen Huang asserts that the performance of the company’s AI chips is advancing at a rate exceeding Moore’s Law, which historically guided computing progress.
“Our systems are progressing way faster than Moore’s Law,” Huang told TechCrunch on Tuesday, following his keynote at CES in Las Vegas. Moore’s Law, coined in 1965, predicted that the number of transistors on a chip would double every two years, doubling chip performance. While the law has slowed in recent years, Nvidia claims its latest data center superchip delivers AI inference performance more than 30 times faster than its previous generation.
Huang attributes this acceleration to Nvidia’s ability to innovate across the full stack: “We can build the architecture, the chip, the system, the libraries, and the algorithms all at the same time,” he explained.
This statement comes amid debates about whether AI progress is plateauing. Nvidia’s chips power AI models at leading labs such as Google, OpenAI, and Anthropic, meaning advancements in its hardware could catalyze further breakthroughs in AI.
Huang also highlights the emergence of three “AI scaling laws” driving progress:
- Pre-training: The initial learning phase using vast datasets.
- Post-training: Fine-tuning models through methods like human feedback.
- Test-time compute: Allowing AI models more time to process and refine answers during inference.
As Nvidia continues to push the boundaries of AI hardware, Huang predicts that the cost of AI inference will decrease dramatically while performance surges, mirroring the cost reductions enabled by Moore’s Law in the early computing era.
(While Nvidia’s dominance in AI chips has made it one of the world’s most valuable companies, Huang’s optimism is undoubtedly aligned with the company’s stake in AI’s continued expansion.)
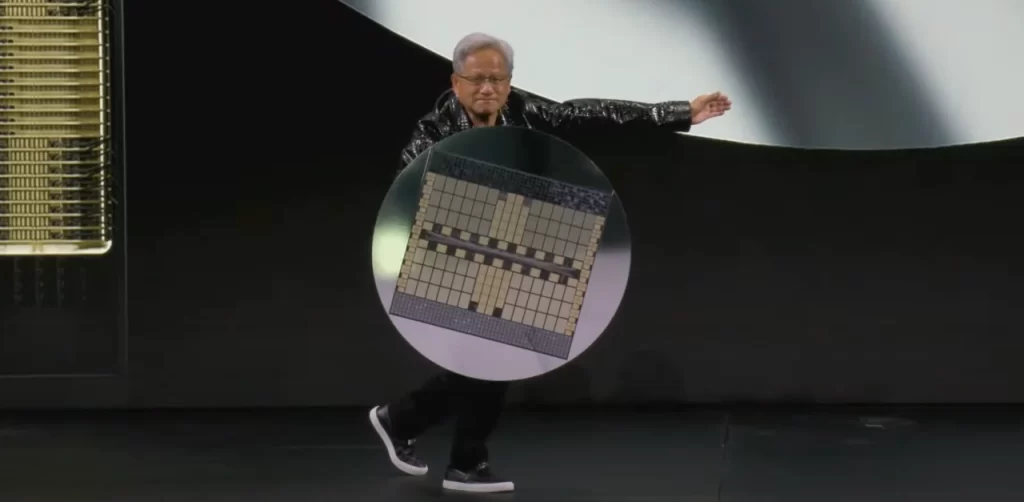
Nvidia CEO Jensen Huang using a gb200 nvl72 like a shield.Image Credits:Nvidia
Nvidia Bets on Cheaper AI Inference with Next-Gen GB200 Superchip
Nvidia’s H100 chips have dominated the market for training AI models, but as tech companies shift focus to inference, questions have arisen about whether Nvidia’s costly chips can maintain their lead. AI models that heavily use test-time compute, such as OpenAI’s o3, face concerns over their high operational costs, which currently limit accessibility. For instance, OpenAI reportedly spent $20 per task using o3 to achieve human-level intelligence scores—equivalent to the cost of a month-long ChatGPT Plus subscription.
During his CES keynote, Nvidia CEO Jensen Huang introduced the GB200 NVL72, a data center superchip designed to address these challenges. The GB200 is 30 to 40 times faster at inference tasks than the H100, promising significant cost reductions for models like OpenAI’s o3, which require substantial computational resources during inference.
“The direct and immediate solution for test-time compute, both in performance and cost affordability, is to increase our computing capability,” Huang told TechCrunch. He emphasized that improved chip performance not only reduces costs but also facilitates advancements in pre-training and post-training, enabling the development of more efficient AI models.
The cost of running AI models has already dropped significantly over the past year, driven by breakthroughs from hardware companies like Nvidia. Huang expects this trend to continue, even as early versions of AI reasoning models remain expensive to operate.
Huang also highlighted the rapid evolution of Nvidia’s AI chips, claiming they are 1,000 times more powerful than their counterparts from a decade ago. This progress far exceeds the pace set by Moore’s Law, and Huang sees no sign of it slowing down.
Nvidia’s latest innovations position the company to lead the charge in making advanced AI capabilities more affordable and accessible, ensuring its continued dominance in the evolving AI landscape.